
AI4ScaDa: AI for Scarce Data - Machine learning and information fusion for sustainable use of laboratory and customer data
Initial situation and problem definition: Companies are currently very keen to harness their data capital for future-proof intelligent products and sustainable value creation. Artificial intelligence (AI) and machine learning (ML) as a discipline of AI represent the key technologies for these companies to analyze and derive value from data. However, the adoption of AI applications by enterprises, especially SMEs, faces hurdles and barriers. In addition, AI methods are predominantly designed for big data and only really develop their potential there. Medium-sized companies, especially SMEs, have much smaller amounts of data available compared to large platform companies, so-called Small Data. Small Data is often defined by sparse data sets (Scarce Data), consisting for example of laboratory data, performance data of machines, personal knowledge (reports) as well as device usage data, which have a high value for the companies, as they contain information about their products and processes as well as their performance and innovation potential. AI development for Small Data applications, especially for Scarce Data, offers great opportunities for these companies and is the focus of AI4ScaDa.
Objective: The AI4ScaDa project pursues both an economic and benefit-oriented objective as well as a methodological objective. The focus is on use cases that are characterized by scarce data and heterogeneous data sources. Use cases of the participating partner companies SAATEN-UNION BIOTEC GmbH, GEA Westfalia Separator Group GmbH and Miele & Cie. KG will confirm the benefit and transferability of the developed solution. The focus is on (i) a product and process design for plant breeding, (ii) a product and process design for separators and (iii) a diagnostic support for networked systems. The goal of all applications is to profitably use laboratory data, coupled with other data sources, for future-oriented innovative products and services. To this end, the project pursues an overarching methodological goal by developing an AI solution consisting of an information fusion and interpretable AI in a modular and generalized form that also supports data collection, e.g., in the laboratory, by means of a feedback loop.
Solution: The AI4ScaDa project relies on information fusion, which is upstream of an interpretable AI. The interpretable AI is realized with ML methods which, due to their structure, have both a high model quality with small amounts of data and a good inherent interpretability. Information fusion prepares and summarizes the heterogeneous data for the ML methods. The interpretable methods aim at providing the user with information about quality, data understanding (extrapolation and interpolation behavior) as well as confidence in addition to result and prediction. This information contributes to more transparency, understanding and acceptance and is also used as a feedback loop to collect more data and add value. The AI solution is built in a modular way through a microservice architecture and uniform interfaces, which ensures transferability of AI4ScaDa into different enterprise structures ensures.
Exploitation of results: The exploitation of results takes place both in the partner companies and via a targeted exploitation strategy. This includes, among other things, the key points of (1) generalization and open interfaces, (2) simple integration, especially for SMEs, (3) best practices, (4) targeted knowledge transfer, and (5) publication of the results in the form of scientific reports and publications. In addition, the realized methodology is implemented on the innovation platform of the Leading-Edge Cluster. Here, a bidirectional exchange takes place by considering existing solutions in the concept phase of AI4ScaDa and finally mirroring the field-tested solutions of AI4ScaDa back to the platform. Furthermore, the solutions of AI4ScaDa flow into the research infrastructures of the research partners and are available there as demonstrators to a wide network consisting of industrial and scientific partners. At the same time, AI4ScaDa is applied in teaching by integrating the methods into lecture contents and by integrating the solutions as a learning platform into the laboratory environments and real environments of the universities.

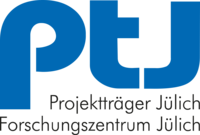