
AVA: Abstraktion von Verhaltensmodellen für Anlagen des Maschinenbaus aus Messungen in verteilten Automatisierungssystemen
Motivation
In automation engineering, methods exist (i) to capture the overall state of production and process plants, and (ii) for the early detection of degradation and anomalies. In this project, these approaches are extended: First, distributed automation systems are used for data collection. Second, new approaches to anomaly detection in computer science such as learning or the automatic parameterization of plant and process models for model-based diagnosis are used.
Projectgoals and researchactivities
To achieve high degree of utilization, and short maintenance periods, signs of degradation should be recognized as early as possible. Today, threshold-based methods often cannot do this. Operators can recognize these behavior changes often too late and this may lead to high maintenance costs and extended downtimes. Here, to overcome this problem, the project uses more complex and more dynamic models of the normal behavior.
The models of the normal behavior are automatically learned based on observations in the operation phase of the system. In this project, algorithms are developed which learn the models in form of hybrid temporal finite automata. The learned models are then used for anomaly detection. During runtime, the forecast of the learned model is compared with the current system observations. For each deviation, an error is signaled.
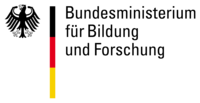
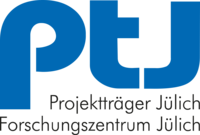